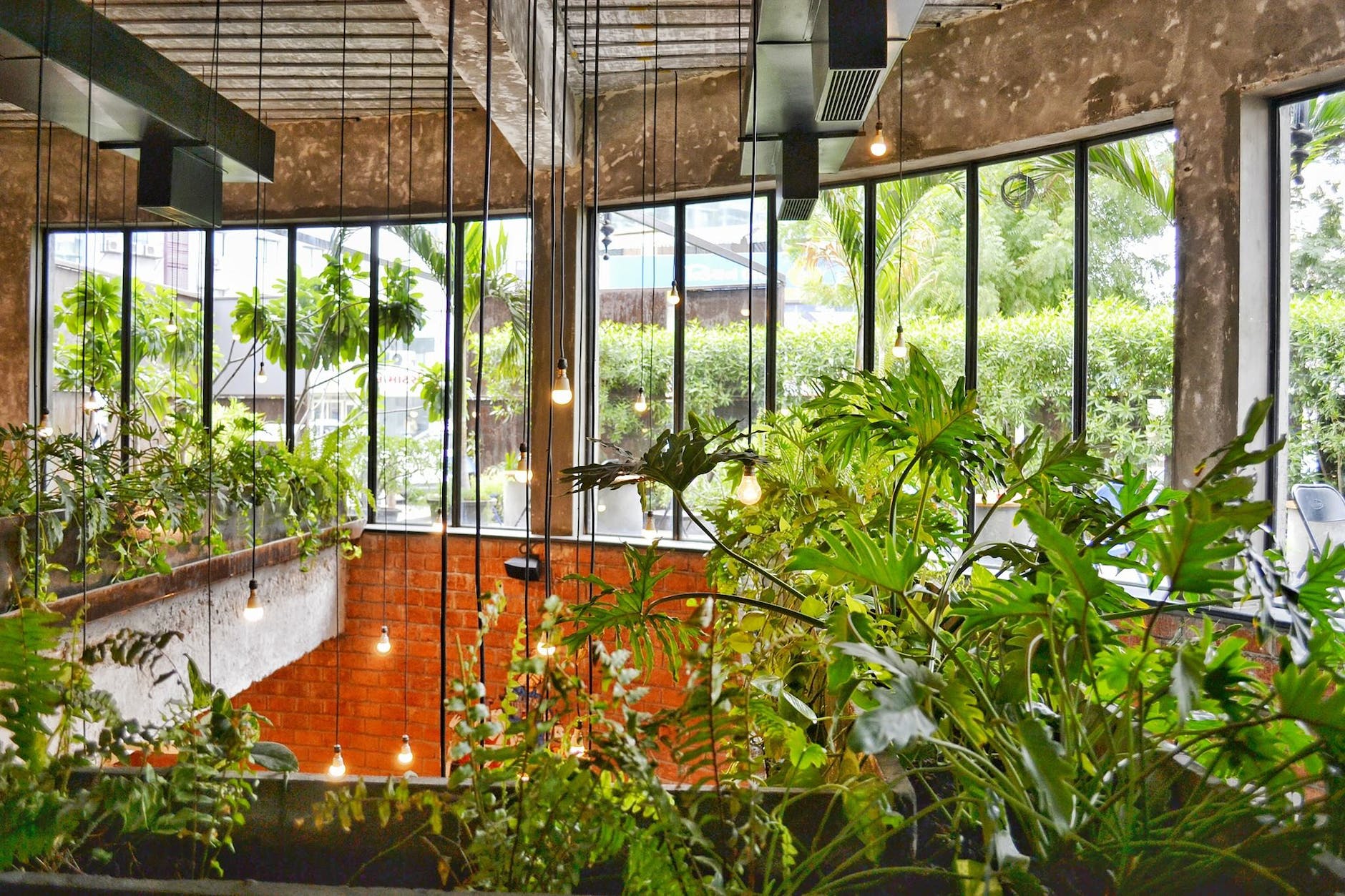
As we continue to embrace the benefits of smart technology, the concept of the Green Intelligent Home is an exciting and promising development in the evolution of smart homes. Our paper, which I presented at the IoTBDS conference in Prague last weekend, explores this frontier.
The possibilities of a world where Green Intelligent Homes are the norm are intriguing, as they offer increased automation, personalization, sustainability, and more. Nonetheless, as with any emerging technology, it is important to be aware of potential risks and implications. These range from security and privacy, manipulation of people, a lack of self-sufficiency, and more.
As the Green Intelligent Home prospect continues to develop, it is essential to stay informed and explore the potential of this technology. In case you are interested in learning more about the Green Intelligent Home or collaborating on related projects, please get in touch.